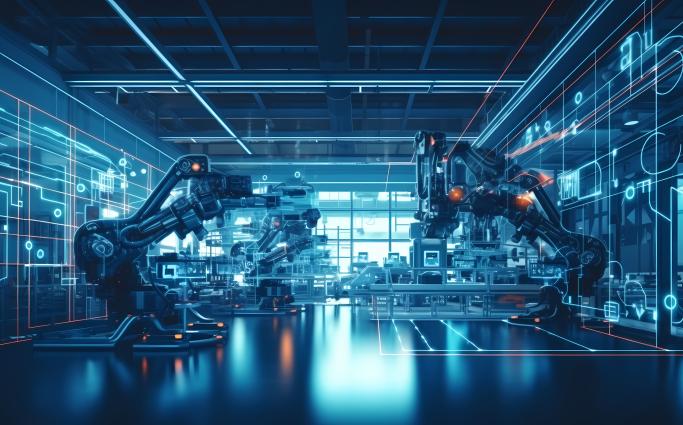
Key Takeaways
Infrastructure is critical for AI advancement. Estimates for data centre chip usage suggest a potential 9x jump in the coming years, from 5.1 million in 2023 to 46 million in 20271.
AI is increasing overall energy consumption, and data centres could account for up to 7.5% of total U.S. electricity consumption by 2030.
Companies are turning to smart grids, software optimisation, edge computing and more to help fill the supply-demand gap.
AI's meteoric rise has the potential to add USD 13 trillion to the global economy by 20302, but its future growth heavily relies on two often-overlooked components: infrastructure and energy. From data centre buildouts to energy-efficient tech, learn how industries are adapting.
Over the past decade, artificial intelligence (AI) has revolutionised entire industries and everyday life. AI adoption rates have soared across sectors, with some estimates suggesting that AI technologies could add USD 13 trillion to global economic output by 2030.
This explosive growth underscores AI’s pivotal role in driving innovation and productivity across the globe. But from our vantage point, two less frequently discussed components hold the key to AI’s future growth: infrastructure and energy.
Why infrastructure is so critical to AI
Infrastructure serves as the backbone for AI innovation and advancement. It provides the computational power, data management capabilities and security required to support the development, deployment and operation of AI solutions. The more we use AI, the more data is generated and processed by AI algorithms, and all that information needs to be securely processed and stored in efficient locations.
- Data centres provide robust storage solutions and efficient management systems, but substantial investment is needed to expand the existing infrastructure. This will allow organisations to handle larger datasets and deploy AI solutions across domains.
- Chips are the backbone of a data centre, able to process data and rapidly calculate billions of results, enabling servers and other electronic devices to function. But to keep pace with current growth of the AI market, some experts think chip demand in data centres could grow by more than 9x over the next few years, from 5.1 million in 2023 to 46 million in 2027.
- With data centres, location matters. Real-time AI applications – such as autonomous vehicles, healthcare diagnostics and industrial automation – require low latency for rapid decision-making. This may require building more data centres to enable faster response times and enhance the performance of AI applications.
Source: Corporate reports, Mercury Research and New Street Research estimates and analysis, as at 4 Jan 2024. 2024-2027 figures are estimated.
How AI is impacting the energy grid
Energy is another key component that can facilitate or hamper the growth of AI. Data centres could account for up to 7.5% of total U.S. electricity consumption by 20303, with some estimates suggesting usage could triple by the end of the decade – from 126 terawatt hours in 2022 to 390 terawatt hours by 20304. Energy consumption directly impacts the operational costs of running AI systems, and generating all that power can have a noticeably negative environmental impact.
Where is all this demand coming from? Much of it is from AI-enabled devices and applications -- such as smart sensors, autonomous vehicles and “internet of things” (IoT) devices – placing additional strain on existing telecommunications networks and power grids. These technologies rely on high-speed internet connectivity and uninterrupted power supply to function effectively.
So, what’s being done? Over the next several years, we expect several trends to shape AI’s power consumption landscape.
- Smart grids can help balance supply and demand in real-time, optimise transmission, and minimise energy losses, leading to a more resilient and sustainable energy infrastructure.
- Advancements in hardware design, such as the development of more energy-efficient processors and specialised AI chips, will lead to significant reductions in power consumption.
- Software optimisation techniques will play a crucial role in minimising power usage without sacrificing performance. Tools and techniques like model pruning, quantisation and low-power inference algorithms aim to be more energy-efficient without compromising accuracy.
- There will also likely be a continued push towards edge computing and on-device AI processing to reduce the reliance on cloud resources and minimise data transfer. This shift will not only improve latency and privacy but also lead to lower power consumption by eliminating the need to transmit data to centralised servers for processing, driving down power consumption in various applications from smartphones to IoT devices.
The bottom line
The growing demand for artificial intelligence is set to reshape the existing infrastructure and energy landscape, which could be an exciting development for investors.
- Infrastructure buildout: Making AI scalable necessitates significant investment. We’re finding opportunities in companies involved in data centre construction, chip manufacturing and network infrastructure development.
- Energy efficiency solutions: Given the rising energy consumption of AI systems, companies are finding new ways to optimise hardware and software. Smart grid technologies can also help meet the energy demands of AI while promoting sustainability.
- Edge computing: Companies involved in developing edge computing technologies, low-power inference algorithms and AI chips for mobile and IoT devices could benefit from the buildout of “edge AI”. This approach not only improves latency and privacy but also reduces reliance on cloud resources, leading to lower power consumption and enhanced efficiency.